[ Fall 2014 ]
As cholera swept through London in the mid-19th century, a physician named John Snow painstakingly drew a paper map indicating clusters of homes where the deadly waterborne infection had struck. In an iconic feat in public health history, he implicated the Broad Street pump as the source of the scourge—a founding event in modern epidemiology.
Today, Snow might have crunched GPS information and disease prevalence data and solved the problem within hours. And in a cellphone text, he might also have sought advice from a certain professor of computational biology and bioinformatics at Harvard School of Public Health, who likely would have spun off dozens of ideas about study design, data analysis and modeling, and interpretation. That’s how John Quackenbush operates.
Boyish-looking at age 52, with long hair, a fashionably scruffy beard, and a wardrobe stocked with jeans, black T-shirts, hiking boots, and a black leather jacket, Quackenbush looks more like an indie band member than someone at the forefront of mapping modern insights into the causes of disease. He has created novel methods of analyzing today’s relentless flood of digitized information that is itself scourge or salvation, depending on how it is harnessed.
“We’re making huge investments in technology to generate data. We’re making huge investments in electronic medical records,” he says. “What’s surprising is not what we’ve done, but what we haven’t done. We haven’t made a parallel investment in tools to make sense of all this information.”
What makes a revolution?
Tucked in a warren of labs and wooden cabinets housing glass flasks, Quackenbush’s scientific lair is a casual repository of digital data, stored on black computer towers lying helter-skelter. It is also an homage to domestic bliss, festooned with photographs of his wife, Mary Kalamaras, an editor and photographer, and his 8-year-old son, Adam.
Mulling the megatrend that big data represent, Quackenbush likens today’s genomics revolution—kindled by the sequencing of the human genome in 2000—to other turning points in the history of science. In the early 17th century, when Galileo built a telescope and pointed it at Jupiter, he found that the planet was circled by moons—an observation that confirmed Copernicus’ theory that the earth revolved around the sun, and thus shuffled humankind’s rank in the cosmic order. Likewise, in the 19th and 20th centuries, experiments conducted at the extremes of velocity and distance enabled physicists to unveil the structure and interactions of nature’s tiniest particles.
In essence, these turning points forced us to reimagine ourselves and the world around us. Today’s genomics revolution will have the same effect.
The science is being driven, in part, by economics. In 2001, it cost about $100 million to figure out the order of DNA nucleotides—the billions of A’s, C’s, G’s, and T’s—in an individual’s genome; and it took months for armies of researchers around the world to generate and interpret the data. By 2009, the cost had dropped to $100,000 and the time required to a few weeks. Today, it costs between $1,000 and $2,000— an easy credit-card purchase—and takes a day or two.
Put simply, genetic sequencing has become a commodity. “We are awash in data. Biology is evolving from being a pure laboratory science into an information science,” says Quackenbush. “And when you look at all the great scientific revolutions, it’s data that drive new ways of thinking about problems. We all have ideas. We all think we know about how the universe operates. But when you start to get empirical data, you realize that your hypotheses aren’t true. In biomedical research, we’ve had a lot of ideas about everything, from ‘What are the origins and evolution of humans?’ to ‘What is the basic nature of disease?’ Genomic data are fundamentally changing the way we think about those questions.”
Amassing data from disparate sources
Indeed, says Quackenbush, big data may represent a treasure trove of potential solutions to countless medical and public health problems. In a few years, researchers will be able to conduct large observational cohort studies that yield whole-genome sequences on hundreds or thousands of volunteers. They could then link the genomic information to diet and lifestyle, health records, environmental exposures, and other data. Once this digitized information is amassed, synthesized, distilled, and analyzed, it could offer clues to how our genetic profiles raise the risk of certain diseases or protect us, and how our genes interact with what’s inside and around us.
“Environmental exposure such as cigarette smoking or obesity have much greater relative risks than almost any genetic factor you can imagine,” says Quackenbush. “But everyone has weird Uncle Bob who smoked until he was 90 and never coughed. Or, on the other hand, a friend or relative who never smoked but developed spontaneous lung cancer at 40.”
Who transmitted infection to whom?
Big data may help scientists detail the spread of HIV more reliably than through contact tracing, which is based on first-person recollections. “Today, we ask people about their sexual partners to track the movement of the infection. Or we collect empirical data and map the flow of disease in social networks,” observes Quackenbush.
“But someday, we will be able to sequence the virus and in that way actually pinpoint who has transmitted the infection to whom, by tracking the mutations that the virus has picked up. Why is that important from a public health viewpoint? Because understanding how the disease is transmitted in networks helps you develop strategies to stop it. Even today, tracking diseases like SARS, MERS, and Ebola involves analyzing combinations of modern molecular and social interaction data.”
Big data might even reveal hidden associations between apparently disparate afflictions. “One of the things I would love to be able to do is look at all the different diseases that co-occur in people,” says Quackenbush. “If we had genetic information, we could combine all that data together to understand if certain genetic risk factors predispose you not to one disease, but to a host of seemingly different diseases.” For instance, a genetic twist in an epithelial cell in the colon that raises the risk for cancer might also raise the risk of asthma or chronic bronchitis in an epithelial cell in the lung. “If we start to see such connections,” says Quackenbush, “we can think about common risk factors and even common therapies.”

“I loved the mad scientists.”
Quackenbush was 5 when he performed his first bold scientific experiment: mixing cleaning chemicals in the bathtub. “That kid is still at the core of who I am,” he says. “When I was little, I watched Batman on TV and I loved the mad scientist villains the best. I came to be excited about science because it involved this process of discovery. I want to understand how things work.”
The quest to understand how things work—and to solve problems by discerning connections—has also been a theme in Quackenbush’s own life. “My father was extremely abusive. We had a lot of domestic violence.” After his parents divorced, he lived with his mother, who worked as a nurse. When he was 12, his father briefly kidnapped him and his sisters. Quackenbush says he hasn’t had contact with his father since, adding, “He was at the far end of the spectrum of acceptable behavior. His antics didn’t create a healthy environment for any of us.
“Would I change any of that? I don’t think I would. The road we take is a big part of who we are. The experiences we have are what make us. All of us face different adversities in our lives, and the challenge is to overcome them. The most important lesson I’ve learned is how to fail.”
The lesson also applies professionally. “As a scientist, if you are working at the edge of your understanding, you are going to come up with ideas that are just plain wrong. If you are a successful scientist, you have to be prepared to quickly figure out why you’re wrong and then try something else. And if you do that enough, you develop an intuition that can help you be wrong less often. But if you aren’t failing, you aren’t trying.”
Physics to biology
Quackenbush’s first scientific passion was theoretical physics. “In physics, we draw conclusions about things we can no longer see and observe. We collect data and plug them into theoretical models. Then we refine those models to see where they break down, so that we can reinterpret the data and build a better understanding of how some particle or force functions.”
In the 1980s, in graduate school at the University of California, Los Angeles (UCLA), he toiled for months on a particularly elusive problem. By the end, he had written a 60-page calculation. “I kept going back to my adviser, who kept telling me it was wrong. The third time, when I went through it and got the same answer, I knew that I was right all along. It was an epiphany. I was sitting in this little office. The weather was dreary. And I had this feeling of sheer joy at discovering a tiny little corner of the universe that no one else knew existed.”
At the time, however, interest in his blissful theoretical corner of the universe was waning. With the Cold War drawing to a close, government funding for physics research dried up. By 1990, Quackenbush’s fascination with high-energy physics had mutated. “I had been on an experiment at Fermilab outside of Chicago. I had just come back to UCLA from weeks— including Thanksgiving—of manning the experiment on the midnight-to-8 a.m. ‘owl’ shift. I walked into my office and was greeted with the news that our funding had taken a severe cut and that, as a postdoctoral fellow, I was expendable. It was a devastating experience. I spent that night feeling like a complete failure. But the next morning, I woke up and asked myself where the most interesting unsolved scientific problems were.”
Helping a girlfriend, who was a PhD student in biology, analyze her data, he discovered a seamless fit between the burgeoning field of molecular biology and his physics training, which had taught him a two-step approach: distill the question to a problem one can solve, then generalize the answer into universal principles.
Quackenbush soon moved swiftly through the most prestigious molecular biology and genomics programs in the country. In 2005, his scientific peregrinations brought him to Boston, with dual appointments at HSPH and at the Dana-Farber Cancer Institute.
At all these posts, the animating impulse behind Quackenbush’s science was transparency. In 2013, he was named a White House Open Science Champion of Change for making open sharing of scientific data a reality. “We don’t publish a paper without ensuring that both the software and the data are accessible, so that other people can reproduce our work,” he says offhandedly. The award committee couched the achievement in grander rhetoric: “Since the Human Genome Project began in the 1990s, new technologies, producing previously unimaginable quantities of data on human health and disease, have been driving a revolution in medicine and biomedical research. John Quackenbush has been a pioneer in ensuring that these data, and the tools needed to access them, are available, accessible, and useful.”
Explaining women’s greater risk of Alzheimer’s
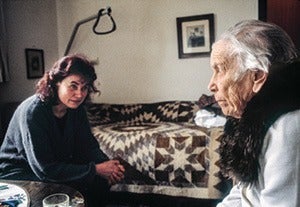
One of the emerging mysteries in medicine is why women and men face different risks for a number of common deadly conditions, from heart disease to chronic obstructive pulmonary disease. Alzheimer’s disease reflects one of the starkest gender imbalances: two-thirds of sufferers are women.
Focusing on Alzheimer’s, Quackenbush and his colleague Kimberly Glass are applying new computational tools to a data set that had been around for more than a decade and had already been extensively analyzed. But they are asking a new question: Are active genetic circuits being switched on and off differently in men and women? What they found was that in Alzheimer’s patients, certain genes were indeed activated differently in men and women—and these genes were highly responsive to estrogen and testosterone. As Quackenbush sees it, “There are subtle hormonal balances that appear to hold the system in check.”
It was a startling discovery, and for Quackenbush it opened up fresh avenues of research. “If the genes activated in Alzheimer’s disease are hormonally responsive, would something like hormone replacement therapy (HRT) in women have a protective effect—or might it actually increase risk? We don’t know.”
To arrive at the answer, he envisions using epidemiological data from large cohorts—such as from the Framingham Heart Study or from the Center for Medicare and Medicaid Services—to tease out whether women who received HRT are at higher or lower risk for Alzheimer’s disease. If HRT is proven to lower the risk of Alzheimer’s, “then we can provide women with the option of considering whether they want to take on the known risks of HRT—an increase in breast cancer—to mitigate the risk for Alzheimer’s. But first we need to conclusively establish the link.”
Deploying big data in this manner may transform the way science is conducted. Rather than dissecting the function of individual genes and then carrying out years of clinical trials to confirm a hypothesis, investigators may simply be able to analyze existing data. According to Quackenbush, “There are certain questions where, if the big data evidence is strong enough, doing the clinical trial may not be practical or even necessary.”
Personalizing medicine
The most immediate application of genomics will likely be in personalized medicine. Even today, genetic profiles are being used to target treatments for everything from breast tumors to heart disease to neuropsychiatric disorders. Quackenbush sees more possibilities. He is currently exploring cancer treatment through a reversed lens: asking whether a tumor’s genetic profile correlates with its size, shape, density, and, most important, invasiveness. If it does, then doctors could potentially determine the genetic profile of a malignancy based on simple CT scan images, which in turn would inform treatment.
“If I can test your tumor for $1,000 and tell you that you’re not likely to respond to a particular therapy that would cost $30,000, that’s a huge public health win,” he notes, “because that money can be used for other potentially effective therapies, or to support other parts of the health care system. And hopefully we can then help you move more quickly to a treatment with a greater likelihood of being effective.”
Quackenbush’s commitment to the data revolution is not merely theoretical. “My grandmother died of Alzheimer’s. I don’t know if she carried an APOE [apolipoprotein E] mutation—which raises the risk of the disease—or not. But I guarantee you that at some point, I’ll be sequenced. From my personal perspective, there is tremendous power in information.”
Like learning a language
For all the popular enthusiasm surrounding big data, the diatribes against it are growing: that it’s noisy and rife with false associations; that it doesn’t necessarily equate to knowledge or understanding; that it doesn’t reflect the real, messy world—dubbed “thick data”; and that it won’t solve complex human problems.
“I would say all of those things are true,” concedes Quackenbush. “Data by itself is not a panacea. But that doesn’t mean we can’t use it. We just need to be smart about how we use it. My experience over the course of my lifetime is that the more information we have, the greater is the opportunity to learn new things. The challenge—and the opportunity—rest in separating meaningless correlations from causal relationships.”
Getting a handle on big data and genomics is like mastering a language, he adds. “There are tens of thousands of words. You can get by just fine with a few hundred. But the subtleties and complexities of what we can convey by using the entire spectrum of the noisy lexicon is part of the joy of being able to speak and communicate.”
Quackenbush clearly revels both in doing the science and in talking about the adventures and misadventures along the way. Hands resting on head, eyes widening, he says, “The most exciting moment is when the data don’t agree with the model. We’re always looking to be surprised.”
—Madeline Drexler is editor of Harvard Public Health
Photo credits: Kent Dayton / HSPH; © REUTERS; © Mikkel Ostergaard / PANOS
Download a PDF of Big data’s big visionary