Congratulations to postdoc Tian Gu, who recently awarded the 2022 Postdoctoral Fellow Research Fund from the Harvard Data Science Initiative (HDSI). The fund incentivizes and supports cross-disciplinary collaboration between data scientists at the postdoctoral level. Dr. Gu works with Dr. Rui Duan and her project is on “A step closer from discovery to clinic: an integrative breast cancer risk prediction tool for African American women.” Abstract: Polygenic risk score (PRS) has shown significant clinical potential for breast cancer risk stratification through aggregated genetic risk effects. Yet, the disparate performance across ancestry groups has hindered PRS from being implemented to refine current risk estimates based on clinal information. Particularly, although African American (AA) women have been suffering from a higher incidence of an aggressive form of breast cancer at a younger age than others, few PRS models have been developed targeting AA women due to their limited representation in large-scale clinical and genomics studies. Moreover, the existing risk prediction models based on clinical information or PRS derived from European ancestry have been found to have poor generalizability in African ancestry. Therefore, we propose to develop an integrative data integration, risk factor detection, and prediction tool that aims to improve the AA women’s breast cancer risk prediction by combining PRS and clinical risk factors, leveraging shared information from multi-ancestry groups, and accounting for population heterogeneity. Our proposal includes (i) using statistical methods to identify and quantify the shared risk factors, both clinical and polygenic, across ancestry groups and further detect the AA-specific risk factors; (ii) using machine learning approaches to develop an integrative breast cancer risk prediction tool for AA women that accounts for clinical and polygenic risk factors, leverages information from other ancestry groups, and quantifies the prediction uncertainties; and (iii) developing a user-friendly platform for health professionals to assess the breast cancer risk in AA women with open-access and reproducible codes.
Postdoc Tian Gu Receives HDSI Funding
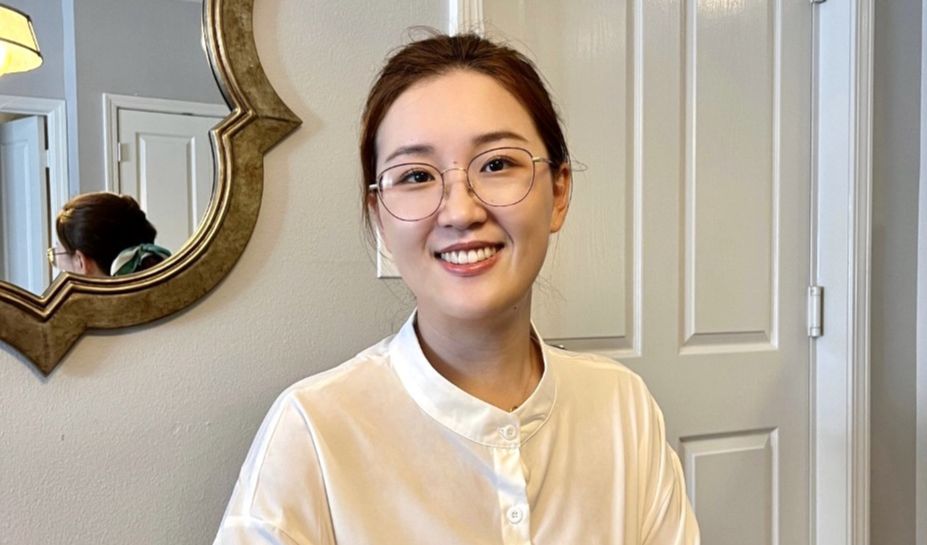